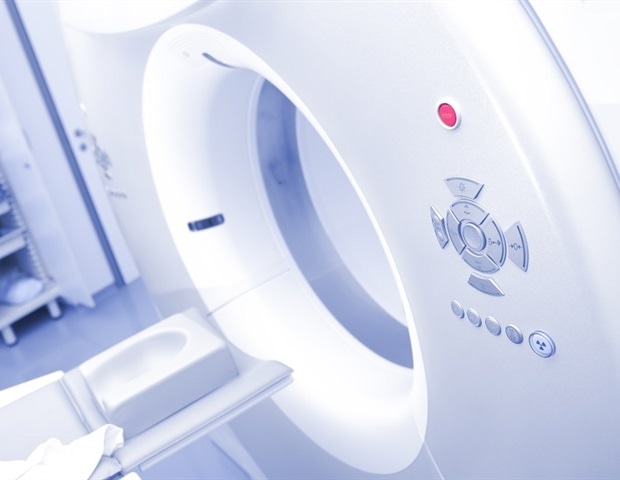
Personalized medicine has stirred the imagination of drugs and therapies that are individually tailored to patients. In the future, there will no longer be a need to worry about side effects, and patients will be screened to identify which treatment will be most effective for them, instead of for the average population. Deep learning is one tool that will be key to realizing personalized medicine.
A new study by Japanese researchers describes a new deep learning tool that will advance personalized medicine for musculoskeletal diseases. The tool can segment individual muscles for a comprehensive model of the musculoskeletal system, which is expected to advance personalized biomechanics.
Accurate measurements of the musculoskeletal system can have a tremendous impact for the extremely ill, like those suffering from ALS or other severe forms of atrophy, to influence the design of rehabilitation devices, and for the extraordinarily gifted, like high-performance athletes who want to take their game to the next level. These measurements come from computed tomography or other imaging modality with which researchers build computer models to study the forces and stresses on muscles and bones.
"Once we have the CT images, we need to segment the individual muscles for building our model," explains Professor Yoshinobu Sato of Nara Institute of Science and Technology (NAIST), Japan, who led the study.
"However, this segmentation was time consuming and depended on expert-knowledge. The figure shows an overview of our system. We used deep learning to automate the segmentation of individual muscles to generate a musculoskeletal model that is personalized to the patient," he continues.
While it is normal to expect a physician to evaluate the images, this adds a level of subjectivity to the diagnosis. The system is especially beneficial for patients in remote areas with limited access to expert orthopedic surgeons, for decisions based on a more quantitative interpretation should improve outcomes.
The method depends on Bayesian U-net architecture.
U-Net is a deep learning framework based on a fully convolutional neural network for the precise segmentation of images. Our colleague, Dr. Yuta Hiasa, extended U-net by combining Bayesian inference to formulate Bayesian U-net, in which uncertainty is associated with segmentation results."The challenge in segmenting individual muscles is the low contrast of the imaging at border regions of neighboring muscles. To test their system, the researchers examined 19 muscles in the thigh and hips. Bayesian U-Net had better segmentation accuracy than other methods including the hierarchical multi-atlas method, which is viewed as state-of-the-art, and did so while reducing the time to train and validate the system by a surgeon.
Professor Yoshinobu Sato, Nara Institute of Science and Technology
"Some pixels in the images had high uncertainty. It was these pixels that especially need confirmation by surgeons," notes Sato.
The researchers thus defined an uncertainty threshold to identify which pixels required human verification.
"Bayesian U-Net learned the musculoskeletal anatomy to create segmentations that would have been created by experts with high fidelity and our collaborator orthopedic surgeon, Prof. Nobuhiko Sugano of Osaka University Hospital, is quite satisfied with this achievement," says Sato.
Nara Institute of Science and Technology
Journal reference:
Hiasa, Y., et al. (2019) Automated Muscle Segmentation from Clinical CT using Bayesian U-Net for Personalized Musculoskeletal Modeling. IEEE Transactions on Medical Imaging. doi.org/10.1109/TMI.2019.2940555.
No comments
Post a Comment