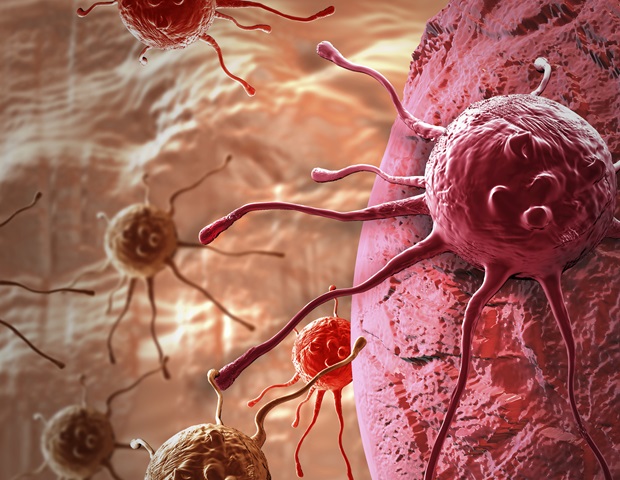
Many cancer patients do not get the opportunity to discuss their wishes for treatment until their illness has progressed too far, when it may be too late to have those discussions with loved ones or physicians. Now, a Penn Medicine-led team has developed an algorithm that flags patients who would benefit most from a timely conversation about their end-of-life goals and wishes, in an effort to begin that dialogue sooner.
In a new study - published today in JAMA Network Open and simultaneously presented at the American Society of Clinical Oncology (ASCO) Supportive Care in Oncology Symposium in San Francisco, CA - researchers found that 51 percent of the patients the algorithm flagged as "high priority" for these conversations subsequently died within six months of their evaluation, compared to less than four percent in the "lower priority" group. These findings suggest the algorithm accurately captures those patients who would benefit most from timely discussions about their goals, values, and preferences for care.
This study is believed to be one of, if not the first, to look into the application of a machine learning algorithm for oncology patients.
On any given day, it's actually pretty difficult to identify which patients in my clinic would benefit most from a proactive advanced care planning conversation. Patients oftentimes don't bring up their wishes and goals unless they are prompted, and doctors may not have the time to do so in a busy clinic. Having an algorithm like this may make doctors in clinic stop and think, 'Is this the right time to talk about this patient's preferences?'"
Ravi Parikh, MD, study's lead author, instructor of Medical Ethics and Health Policy at the University of Pennsylvania and a staff physician at the Corporal Michael J. Crescenz VA Medical Center
The researchers applied three different predictive models to 26,525 patients receiving outpatient oncology care at two hospitals within the University of Pennsylvania Health System. Each used information commonly available in patients' electronic health records: demographic characteristics such as gender and age, standard comorbidity data such as whether a patient has high blood pressure, and laboratory and electrocardiogram data.
Compared to other machine learning techniques, such as "gradient boosting" and "logistic regression," the "random forest model" the team built in this study led to the best predictive results: Roughly half of the high-risk patients died within six months and almost 65 percent died approximately a year-and-a-half later (compared to 7.6 percent of low-risk patients). When 15 oncologists were surveyed, they agreed that 60 percent of those identified by the algorithm as "high risk" were definitely appropriate for immediate conversations about their wishes.
This algorithm was created with help from a team of Penn Medicine researchers who also developed a different algorithm for similar purposes, called Palliative Connect. That system is used to trigger consultations with Palliative Care staff, and was recently found to be particularly effective, increasing consultations by 74 percent. But the study led by Parikh is different in that it is targeted at increasing conversations between patients and the oncologists themselves in the outpatient setting.
"We're excited about the scalability of this decision support method for providers, and not just in oncology," Parikh said. "Our process of using machine learning to flag high-risk patients in real time is broadly applicable, and our approach risk-stratifies patients in a usable way that just hasn't been available to us before."
Now that the algorithm has shown promise, Parikh said that it is being implemented at a medical center that was not part of the original pilot for this study. There, researchers are testing whether identifying the best patients for conversations actually does prompt the doctors to initiate those discussions. The researchers are currently working on a randomized controlled trial involving around 100 clinicians that will last between three and six months.
"Initial data from our pilot suggest that using the algorithm can boost these conversations," Parikh said.
University of Pennsylvania School of Medicine
Journal reference:
Parikh, R.B, et al. (2019) Machine Learning Approaches to Predict 6-Month Mortality Among Patients With Cancer. JAMA Network Open. doi.org/10.1001/jamanetworkopen.2019.15997.
No comments
Post a Comment